AI-driven nutritional assessment of seed mixtures enhances sustainable farming practices
Cultivating seed mixtures for local pastures is an age-old method to produce cost-effective and balanced animal feed, enhancing agricultural autonomy and environmental friendliness in line with evolving European regulations and organic consumer demands. Despite its benefits, farmers face adoption challenges due to the asynchronous ripening of cereals and legumes and the difficulty in assessing the nutritional value of heterogeneous seeds. Current practices rely on informal, empirical methods, and a proposed solution is to develop a mobile app or online service, similar to Pl@ntNet, for automated nutritional evaluation of seed mixtures, encouraging farmer participation and database enrichment. However, this requires overcoming agricultural and computer vision challenges. Overcoming these, along with optimizing deep neural network models and loss functions, remains a critical Research focus to make this sustainable agricultural practice more accessible and efficient.
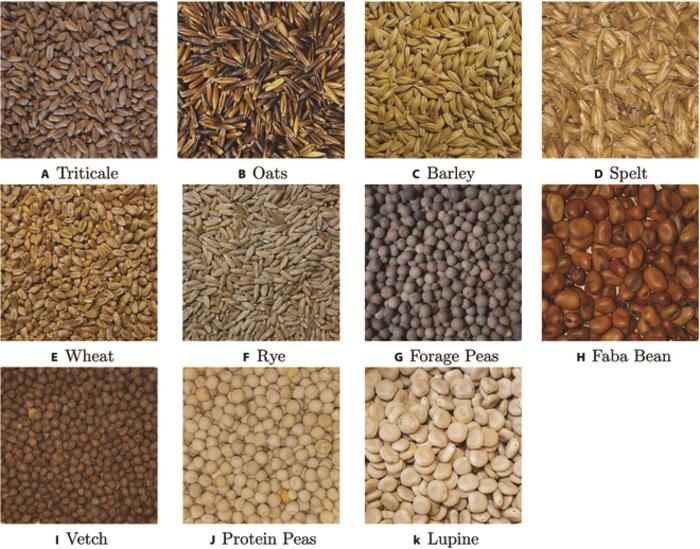
Plant Phenomics
In November 2023, Plant Phenomics published a research article entitled by “Estimating Compositions and Nutritional Values of Seed Mixes Based on Vision Transformers ”.
This research presents a novel approach using Artificial Intelligence to estimate the nutritional value of harvested seed mixes, aiming to assist farmers in managing crop yields and promoting sustainable cultivation. A dataset of 4,749 images covering 11 seed varieties was created to train two deep learning models: Convolutional Neural Networks (CNN) and Vision Transformers (ViT). The results significantly favored the ViT-based BeiT model, which outperformed the CNN in all metrics, including a Mean Absolute Error (MAE) of only 0.0383 and a coefficient of determination (R2) of 0.91. Data augmentation techniques and model size variations further refined performance. Although larger models offering some improvements, the base version of BeiT proved most efficient in terms of balance between performance and computational resources. The study also explored loss functions, finding that the classical KLDiv loss outperformed the Sparsemax variant. Detailed analysis by seed type revealed distinct performance across categories, with models generally excelling in recognizing barley, lupine, rye, spelt, and wheat, while facing challenges with vetch and oats. Aggregating predictions from multiple images of the same mix significantly improved robustness and accuracy. The research culminated in the development of "ESTI'METEIL" (https://c4c.inria.fr/carpeso/), an open-access web component that allows users to estimate seed composition and nutritional value from images. This tool demonstrates the practical application and potential of the research for real-world farming scenarios.
In conclusion, the study effectively applied advanced deep learning techniques, particularly the self-supervised BeiT model, to the agricultural challenge of estimating the composition of seed mixtures and their nutritional values. The research not only showed promising results with a high R2 score but also provided a practical tool for farmers, marking a significant step towards more sustainable and informed agricultural practices. Future work will aim to improve data balance and explore synthetic image generation to further improve model performance and practical applicability.
Most read news
Other news from the department science
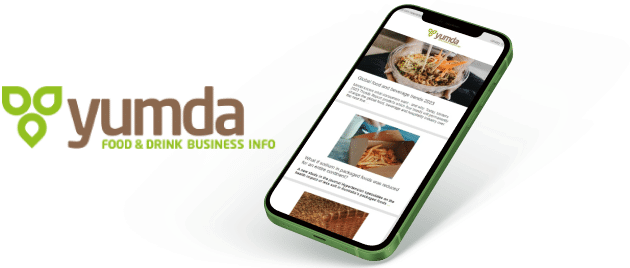
Get the food & beverage industry in your inbox
From now on, don't miss a thing: Our newsletter for the food & beverage sector brings you up to date every Tuesday and Thursday. The latest industry news, product highlights and innovations - compact and easy to understand in your inbox. Researched by us so you don't have to.